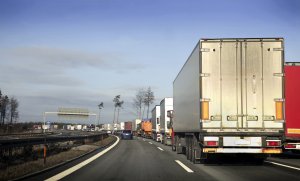
Truck traffic is one of the most important inputs for designing pavement structure and analyzing performance, according to the study. Photo: ©Shutterstock.
The development of this traffic data can be used to generate more accurate predictions of pavement life and performance in the region, researchers say.
Roadways across the United States experience a lot of stress everyday as millions of vehicles drive over them, connecting friends and families, keeping our economy going, and providing access to numerous services.
If roads are to be designed to survive all of this traffic wear-and-tear, it is important that both traffic volume and vehicle/axle weights are known. But, no two roadways are the same and as a result they each require specific designs to support their specific traffic loads.
A new paper co-authored by Center for Advanced Infrastructure and Transportation (CAIT) affiliated researchers, “Evaluation of Clustered Traffic Inputs for Mechanistic-Empirical Pavement Design: Case Study in New Jersey,” analyzed traffic clusters in the state to help characterize traffic data—and more specifically tailored data—into mechanistic-empirical pavement design to better inform future predictions and building methods in the region.
Transportation Research Record: Journal of the Transportation Research Board recently published the paper, which is based on the study conducted as part of the Pavement Support Program sponsored by the New Jersey Department of Transportation (NJDOT).
Dr. Hao Wang, an associate professor in the Department of Civil and Environmental Engineering at Rutgers and a CAIT-affiliated researcher led the study. Dr. Thomas Bennert, an associate research professor at Rutgers and the principal investigator of the Pavement Support Program contributed as a co-author alongside Abbas Jasim, who received his Ph.D. from Rutgers in May 2018 and is now a faculty member at Al-Mustansiriya Universitya in Iraq.
In the United States, many state DOTs have started using the new generation of pavement-design methods based on the Mechanistic-Empirical Pavement Design Guide (MEPDG).
MEPDG uses mechanistic pavement analysis to determine how pavement materials will respond to different environmental conditions and traffic loading—two important variables linked to pavement performance. It can run simulations on pavement to determine when pavement distresses, such as cracks or ruts, reach terminal thresholds. This alerts engineers to when pavement will reach the end of its service life.
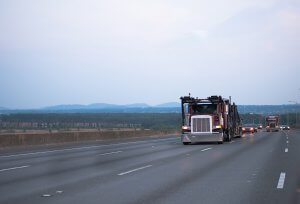
Researchers said they collected truck-traffic data from 76 Weigh-in-Motion (WiM) sites across the state. Photo: ©iStock.
“In order to use MEPDG accurately for pavement design, each state needs to develop their own local calibration data. Truck traffic is one of the most critical inputs in the design of pavement structure and analysis of pavement performance,” Wang said.
The study started with gathering multiple years’ worth of truck-traffic data from the 76 Weigh-in-Motion (WiM) stations across the state of New Jersey. With that, statistical analysis was first conducted to examine both directional and temporal variations within the traffic data.
Researchers then conducted hierarchical clustering analysis to identify the optimum clusters of traffic data in New Jersey. They found three optimum clusters for axle load spectra, vehicle class distribution, and axle/truck ratio, respectively.
Wang said this will enable NJDOT staff to characterize truck-traffic patterns for pavement design at the locations without WiM stations.
Next, to evaluate the accuracy of traffic clusters on pavement design, the researchers considered four traffic input scenarios: site-specific, cluster, statewide average, and default. They found that for 10 selected WiM sites, cluster-traffic data generated the closest predictions of pavement life when compared to site-specific data; while default traffic data yielded the highest error.
“The development of traffic clusters provides the right traffic data for generating accurate predictions of pavement life and performance.” Wang said.
Going forward, the research team is performing local calibration of pavement material properties and performance prediction functions for full implementation of MEPDG in New Jersey.